Introduction
Nearly a third of all patients who suffer from epilepsy experience seizures despite the presence of advanced epilepsy management tools (Bethesda National Institute of Neurological Disorders and Stroke 1). Many researchers agree that the development of advanced techniques to detect seizures before they happen is an effective way for customizing epilepsy therapies to different groups of patients (Watson 1). Similarly, such methods may play an important role in preventing accidents. However, the process of predicting seizures before they happen has not characterized epilepsy management for many decades because doctors always believed that it was impossible to predict seizures because of their randomly occurring nature (Scaramelli & Braga 246; Bethesda National Institute of Neurological Disorders and Stroke 1). However, recent advancements in medical research studies have shown that it is possible to predict seizures from the analysis of different brain and nerve cell patterns, which vary uncontrollably during a seizure. When experiencing a seizure, a patient could manifest different symptoms that include convulsions, rigid muscles, and unconsciousness. Such episodes could lead to fatalities or serious injuries when patients are alone. This is why it is important to predict seizures before they happen to allow patients and caregivers to prepare for such episodes. This chapter delves into explaining the working hypothesis of the mobile epilepsy predictive system, which is an instrument for helping patients to predict these seizures. However, before doing so, it is, important to clarify two terms that lead to confusion regarding this process – prediction and detection.
Prediction vs. Detection
Although there are many volumes of literature that explain what happens during the pre-ictal stage (before a seizure), the terminology is not uniform (Scaramelli & Braga 246). There has been confusion regarding the use of prediction and detection techniques during the management of epilepsy cases. Although many researchers use the term interchangeably, they do not mean the same thing. Prediction refers to the identification of seizure before it occurs (before some measure of ictal manifestation). Comparatively, detection occurs when identification of a seizure occurs at its initial stage. The Bethesda National Institute of Neurological Disorders and Stroke (1) says that although this distinction is simple, it is not always clear to many people. The confusion often occurs through different manifestations of EEG data (Binder 247). For example, data obtained through EEG changes may represent true pre-ictal states, but at the same time, they may denote the earliest manifestations of a seizure. To demystify some of these issues, Binder (247) proposes the development of a conceptual model that would use different intervention strategies for highlighting the differences between prediction and detection processes. Furthermore, he argues that this approach will show that the prediction of seizures can only occur during the pre-ictal period (Binder 247). This is the time when “when either the clinical, electrographic and/or physiological milieu represents a risky state where the likelihood of progression to a seizure is high” (Binder 247). Haut (276) says it is unwise to propose the use of different interventions at this stage because they would be pre-emptive. However, many researchers do not always accept this suggestion because detection usually occurs at the earliest physiological detection of an ictal state (when a seizure starts occurring) (Scaramelli & Braga 246; Bethesda National Institute of Neurological Disorders and Stroke 1). Such detections may occur before any EEG manifestation. In line with this observation, Lasemidis and Shiau (616) say that any intervention introduced at this stage would be abortive.
Types of Detection and Prediction Methods
Lipton (925) says that many researchers have tried to classify different types of epilepsy detection and prediction methods. The most common groups are the linear and nonlinear methods. For example, variance-based and correlation-based methods for epilepsy prediction are linear methods (Lasemidis & Shiau 617). With the exception of the simple power spectrum-based method, Schulze-Bonhage (88) believes that all other seizure prediction and detection methods are non-linear. According to Sackellares (55), researchers could also classify prediction and detection techniques according to the types of EEG used. For example, one category of seizure prediction is the scalp EEG method (Lipton 926). The iEEG is also another category of prediction methods (Lipton 925). Although researchers commonly use this classification to understand different types of epilepsy detection and prediction techniques (Bethesda National Institute of Neurological Disorders and Stroke 1), this paper did not adopt this classification because the mobile epilepsy predictive system is a non-linear technique.
How the Mobile Epilepsy Predictive System Works
Based on recent advancements in medical technology, researchers developed the electroencephalography (EEG) which can detect rapid changes in brain activity before a seizure occurs (Scaramelli & Braga 246). It does so by measuring electrical activity in a patient’s brain, which is a possible sign that a patient may be about to experience a seizure. Conventional methods of measuring brain wave patterns involve attaching electrodes to the scalp, which turn electrical signals into readable patterns (Scaramelli & Braga 247). For a long time, experts have used EEG technology to diagnose seizures before they happen (Lasemidis & Shiau 618). However, this process has often resulted in disastrous consequences, especially when people lose their lives or sustain injuries after a seizure. Therefore, there has been a new push to detect seizures before they happen. This push yielded to further advancements in technology that allowed researchers to use the EEG to do so. In this regard, it has been able to detect abnormal brain patterns, which is a possible sign that a patient could be about to suffer a seizure.
The challenge with EEG is its practicality because it is impossible for epileptics to carry around an EEG machine to know when their next seizure would occur (Lasemidis & Shiau 618). Instead, experts have embarked on renewed efforts to make the technology work by using portable devices, such as smart-phones, which could integrate the EEG technology (Bethesda National Institute of Neurological Disorders and Stroke 3). Such devices should detect abnormal brain waves and relay the same to an electronic device. Heart rate and breathing changes are common physiological symptoms that these devices use to detect changes in the nervous system, to predict when the next seizure could occur (Watson 7). To come up with an accurate understanding of when the next seizure would occur, the EEG uses mathematical formulas to calculate the probability of a seizure occurring. These advanced detection methods used complex algorithms that infuse future computation techniques for detecting seizures. Some common methods used to detect seizures include the scalp and intracranial EEG. Other methods include electrocardiography, accelerometry and motion sensors (Watson 7). This chapter advances the use of the mobile epilepsy predictive system as another method for detecting epilepsy. This method is unique to other tools for detecting epilepsy because it is non-invasive. Its efficacy in doing so comes from its ability to detect a seizure, 40 minutes before it occurs. The instrument also allows a patient to know of an attack through a smart-phone.
The working hypothesis of this predictive instrument has three main stages. The ability of a headset to detect brain waves is a common characteristic of the first stage. The second stage involves the relay of the brain waves (through Wi-Fi data) to a mobile device, personal computer (PC) or tablet. These devices integrate with other stages of the instrument through a signal relay process that raises an alarm to the user, 40 minutes before a seizure occurs. The diagram below illustrates these steps
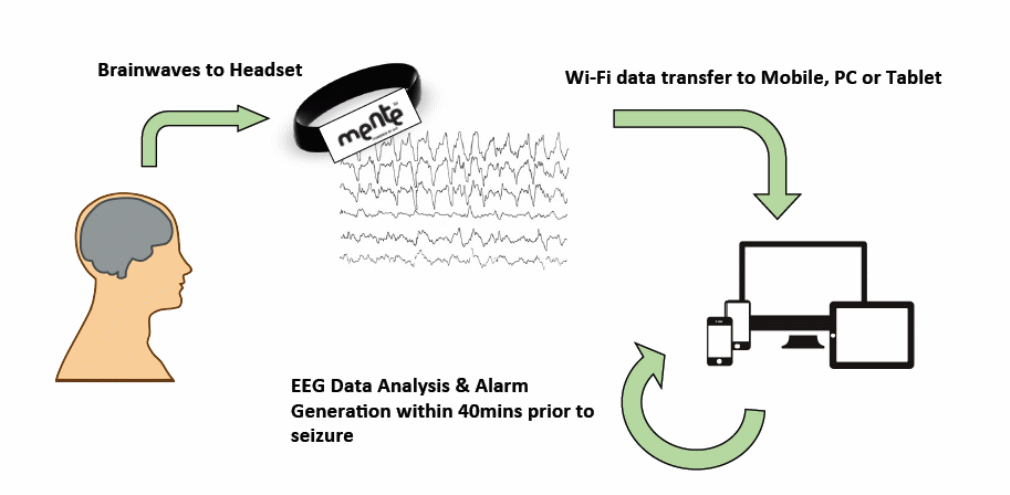
The third stage of the above model is the time between the inter-ictal stage and the pre-ictal stage. The latter stage lasts for 40 minutes as described earlier.
Signal Processing
There have been many developments in the field of processing signals for purposes of seizure prediction (Lasemidis & Shiau 618). For example, there have been several discrete transformations and signal decomposition techniques that have revolutionized how researchers process signals from EEG data (Scaramelli & Braga 247). In line with these developments, the discrete Fourier transform (DFT) has emerged as a popular signal processing technique. Similarly, the discrete wavelet transform (DWT) technique has cut a name for itself as a popular method for processing signals (Scaramelli & Braga 247).
Ictal Stage
The inter-ictal state refers to the period between seizures. It also refers to periods between convulsions. According to Eftekhar (43), many epileptic patients have inter-ictal periods that correspond to 99% of their lives. Experts use statistics from the inter-ictal period to diagnose epilepsy (Schuyler 75). They do so because the EEG will only show small inter-ictal spikes, which are insufficient in detecting, or diagnosing, epilepsy. EEGs also show mere abnormalities (subclinical seizures) that most experts cannot use to diagnose the condition. For example, some EEG discharges only refer to abnormal waveforms that have little, or no, association with seizures, or convulsions, that occur from epileptic symptoms (Bethesda National Institute of Neurological Disorders and Stroke 4). When a seizure occurs (inter-ictal stage), it could last for up to two minutes as described in the diagram below
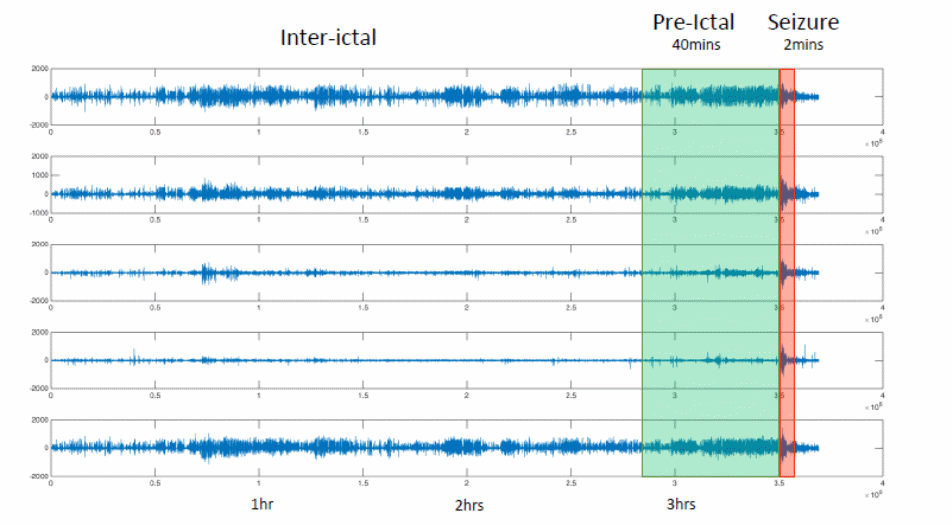
The lag between the inter-ictal and pre-ictal period depends on several factors. According to Scaramelli and Braga (246), understanding these factors is a matter of understanding what somebody is looking for. The mobile epilepsy predictive system chooses to focus on two things – finding the evidence and learning that algorithms find the evidence (Scaramelli & Braga 246). The first stage involves understanding that transformation evidence of the pre-ictal state involves transforming pre-ictal evidence into EEG features. The last stage involves designing tentative features and allowing the learning process to establish if it is evidence or not. The learning algorithm works as shown below
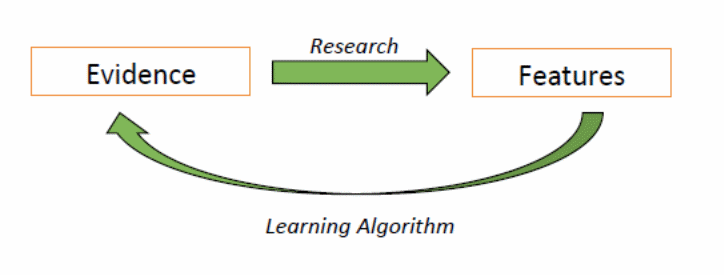
Although the above diagram represents the learning algorithm of how researchers could use evidence to describe unique features of analysis, Ozdemir and Yildirim (3) say that the body’s transition into the inter-ictal stage is unclear. Nonetheless, some researchers claim that the synchronization of a large neuronal population by inhibitory inter-neurons could help demystify some of the unresolved issues (Arizona State University 8). Studies designed to investigate the transition from the pre-ictal stage to the inter-ictal stage have involved humans and animals alike. For example, researchers have used slices of rat hippocampus’s brains to investigate this issue (Fricker 559). Their studies have revealed that GABA actions could be inhibiting (Arizona State University 8). “Previously it was shown that the hyper-excitable state during transition to seizure could be a consequence of alterations in glutamatergic and/or GABAergic synaptic input in both animal and human tissues” (Zhang and Koifman 2499). This statement led many observers to believe that inhibitory and excitatory synaptic transmissions could potentially affect how patients transition from the pre-ictal state to the inter-ictal state. However, several issues still cloud this belief. For example, researchers still do not understand the extent that excitement and inhibiting factors affect the transition between these two states (Arizona State University 8). They also do not understand the mediating mechanisms across the two stages. Lastly, Elsevier (9) says there is little knowledge to explain whether transitions are cell-type dependent or not. Some researchers have strived to explain most of these issues. For example, Watson (3) investigated the relative contributions of synchronized GABAergic inputs in the process and found that the shifting balance between excitatory and inhibitory inputs affected the transition between the pre-ictal and inter-ictal stages. Zhang and Koifman (2500) did a separate study to investigate the same issue using glutamatergic inputs and their functional roles during ictogenesis and found out that inhibitory GABAergic IPSPs and IPSCs often disappear (lessen) during the pre-ictal stage. This process happened because there was a lower GABA release from presynaptic terminals (Amy 42). Relative to this discussion, Zhang and Koifman also “found that this synaptic mechanism of transition occurs in CA3 non-fast-spiking (non-FS) and FS interneurons and pyramidal cells” (2500).
EEG Milieu in the Pre-ictal State
The term pre-ictal state emerged in the early 1970s, when researchers struggled to find new ways of detecting and preventing seizures (Drury 9). In line with this goal, the same researchers used surface or intracranial electroencephalography (EEG) to gather information about seizures. Recent studies have improved the use of computational algorithms to predict and detect seizures. Particularly, researchers have used them to analyse the characteristics of the pre-ictal state using the modalities derived from the EEG (Drury 9). Recent attempts by researchers to improve the quality of information obtained from EEG modalities have mainly followed two distinct strategies. The first strategy involves improving the detection algorithms for predicting when imminent seizures are likely to occur (Neapolitan 15). The second strategy involves improving the EEG spatial and temporal resolutions (Neapolitan 15). These advanced algorithms are supposed to detect patterns that occur in the EEG. Information obtained in this way is supposed to help gather information about impending, or early, seizure activities. At a later stage, they are supposed to provide a trigger for alerting a patient about an impending seizure. In line with this trend, investigators have used different mathematical models to investigate EEG trends (Scaramelli & Braga 246; Neapolitan 15). Their attempts have mainly strived to predict the onset of seizures or muscle convulsions. Despite the numerous research studies conducted on this topic, Haut (276) says there is no better algorithm method that predicts seizures better than chance.
The second strategy for learning EEG patterns involves improved spatial and temporal EEG sampling. Developments that have occurred in this sector have come from advancements in engineering techniques and the use of new monitoring tools for the pre-ictal stage (Bethesda National Institute of Neurological Disorders and Stroke 1). For example, recent advancements in electrode designs have helped researchers to improve spatial sampling. Indeed, such advancements have helped to discover new micro-seizures (Lasemidis & Shiau 620). In particular, individual microelectrodes have been instrumental in helping to make such discoveries. Recent advancements in temporal sampling have also had the same impact because they have helped researchers to detect high frequency EEG oscillations (Lasemidis & Shiau 619). Such information is useful in predicting the onset of seizures.
According to Reynolds (27), new technological developments and advancements in algorithms will improve the quality of information pertaining to understanding the onset of seizures and muscle convulsions. Particularly, they would explain how these effects occur in the brain. Such advancements are important because available information quality has several drawbacks (Lasemidis & Shiau 620). For example, as Hughes and Feldmann (201) observe, many EEG systems have poor spatial resolutions. This makes them have poor resolutions for detecting micro-seizures (this limitation elevates the importance of using invasive electrodes). Besides having poor spatial resolutions, many EEG systems also have poor temporal resolutions, which incapacitate them from detecting HFOs (Reynolds 27). These limitations have elevated the need to develop EEG systems with high spatial and temporal resolutions. In line with this observation, Dzhala (7873) claims that current paradigms require offline retrospective analysis to allow researchers to have a more reliable metric for predicting seizures and convulsions before they occur. This recommendation comes from the errors reported from inconsistencies in detection rates that emerged from previous human trials where patients had recording electrodes implanted in their bodies (Amy 1; Beenhakker (612). Cohen (485) and Binder (247) claim that they have assessed most of the issues discussed here and recommend “that a more important conceptual problem is that the synchronized neuronal discharges which EEG measures are the end result of a preceding physiologic cascade, making early seizure detection or seizure prediction difficult with EEG modalities” (Binder 247). As researchers still ponder on whether to adopt these recommendations, or not, no real-time methods of EEG analysis could accurately predict when a seizure would occur (Dzhala 7873). However, the introduction of the mobile epilepsy predictive system is a useful step towards this direction (formulating an accurate and real-time instrument for predicting the onset of a seizure).
Clinical milieu during the Pre-ictal state
Researchers have often struggled to find the correct understanding of a clinical pre-ictal state because they use different data to describe this category. For example, some of them argue that constellations of symptoms provide the best data for describing this stage (Schuyler 75; Scaramelli & Braga 248). Others argue that the presence of precipitants provide the best data for describing the pre-ictal state, especially because they are more likely to provide a clearer likelihood of an imminent seizure (Lasemidis & Shiau 619). Cohen (7) says that many patients have often known that they are bound to have a seizure, hours or even days before the seizure occurs. However, tests show that such characteristics are implicit to specific cases because studies have shown that some patients have unique characteristics that allow them to know of an impending seizure (Bethesda National Institute of Neurological Disorders and Stroke 4). Therefore, using findings from such patients is unreliable. Furthermore, the data obtained from research that includes such respondents is vulnerable to recall bias. Therefore, the best type of data to use is that which includes information from a large cohort of respondents. The same data should consist of time-stamped information that researchers have collected over long periods. Many studies have collected information from this area, but few of them meet these criteria (Bethesda National Institute of Neurological Disorders and Stroke 4).
The presence of prodromal or premonitory symptoms in epilepsy patients is becoming a new centre of focus for many researchers because these symptoms could provide sufficient data to describe a clinical milieu in the pre-ictal stage (Scaramelli & Braga 246). “Both questionnaire studies and prospective diary studies have identified symptoms such as irritable mood, headache, dizziness, visual changes and concentration difficulties, to name a few, as being reliably prodromal” (Binder 247). However, this finding is contestable because some researchers have opposed it (Cohen 7; Schuyler 75). For example, a recent electronic study contained data obtained from a seizure prediction workshop, which showed that some symptoms are not reliably prodromal (Schuyler 76).
According to Neapolitan (15), precipitating factors are a fairer metric for describing the clinical pre-ictal milieu. He says so because they believe that these factors relate to a time when there is a high risk of seizures (Neapolitan 15). Such precipitated factors may vary according to patients’ demographics. However, the most common factors to consider are stress, sleep, and menstrual status (Neapolitan 15). Litt (22) reports that the ability of patients to self-report a seizure depends on their ability to be aware of these precipitants and the associated prodromal features. Nonetheless, intriguing, clinical research studies have not predicted pre-ictal milieu with the sensitivity it deserves. For example, pre-emptive pharmacological trials require sensitive predictions of pre-ictal milieus (Litt 22). According to Drury (9), this study area is important because if unique prodromal characteristics of predictive symptoms characterized the pre-ictal period, understanding them would enhance ongoing efforts to develop effective instruments for understanding the pre-ictal state.
Physiological Milieu of the Pre-Ictal Stage
Based on the failure of some researchers to predict the onset of seizures using the EEG methods, experts have looked outside the EEG to find ways of giving them specific and sensitive EEG data (Scaramelli & Braga 246; Neapolitan 15). In line with this quest, many of these experts have undertaken animal studies to understand the neurochemical, histological, and behavioural properties of acquired epilepsy (Scaramelli & Braga 246; Neapolitan 15). Despite the nobility of this effort, many of these studies are still unable to predict what happens to a patient’s brain right before the ictal stage. Based on this challenge, many of the available studies have only focused on identifying the physiological processes that occur before the ictal stage (Bethesda National Institute of Neurological Disorders and Stroke 1). Several experiments, such as the 1989 rat hippocampus study revealed that electrical resistance often manifests in the rodents before a seizure occurs (Fricker 559). This resistance often occurred through tissue resistance. The process is synonymous with decreased extracellular space during the ictal period (Bethesda National Institute of Neurological Disorders and Stroke 1). Researchers have extrapolated these findings and applied them to people. They have suggested that a reduction in brain extracellular space could be an indication of an impending seizure (Fricker 559). In a different set of studies, a separate group of researchers (Dudek’s group) found that the reduction in brain extracellular space (ECS) could increase through hypoosmolar treatment (Fricker 559). They say so because they found that the treatment caused epileptiform bursting in the CA1 region of rat hippocampal slices (Fricker 559). Nonetheless, Lopantsev (352) reveals that mannitol could reverse this effect.
The above findings have further prompted the creation of new questions in this area of research because researchers, such as Carney (94), ask if the constriction in the extracellular brain space occurs in vivo. To answer this question, the Arizona State University, says that “Early pioneering studies of ECS parameters using the iontophoretic TMA+ method demonstrated significant constriction of the ECS during seizure activity in vivo, but the temporal onset of ECS constriction relative to seizure onset remained unclear” (56). To explore some of these issues, Shiau (616) and Lopantsev (352) have taken upon themselves to conduct further tests using the cortical fluorescence recovery technique. They used photo bleaching to establish the real-time extracellular space that occurs before a seizure happens. They then applied this model to the PTZ model of general seizures and found that “The photo-bleaching technique involves in vivo loading of fluorescein-dextrans into the extracellular space (ECS) of intact brain, followed by laser-induced spot photo-bleaching and measurement of fluorescence recovery using confocal optics” (Binder 248). Similar to the processes observed during cytotoxix exema, changes in the extracellular space brought further changes in diffusion rates within the brain cavity (Shiau 616; Lopantsev 352). Stated differently, there has been slowed diffusion rates associated with this process. The Bethesda National Institute of Neurological Disorders and Stroke (2) has used these findings to argue that the decreased ECS diffusion could be a predictor of an impending seizure. These findings have shown the proof of concept concerning the use of water movements to detect seizure activity (Shiau 616; Lopantsev 352).
Similar to the above studies, Binder (247) has also undertaken independent research to predict the onset of seizures through electrical impendence monitoring. In this study, the researcher treated animals using picrotoxin and kainic acid (Binder 247). In some cases, he also used fluorocitrate on the animals. Similar to the 1989 findings of Traynelis and Dingledine’s (cited in Carney 94), Binder (247) found small instances of electrical tissue impedance in the animals before the onset of a seizure. He also found that the electrical tissue impedance increased with an increase in non-ictal high-frequency EEG activity (Binder 247). According to this analysis, there may be a direct correlation between ECS changes and pre-ictal HFOs. Similarly, there may be a direct correlation between detectable tissue impedance and pre-ictal HFOs (Bethesda National Institute of Neurological Disorders and Stroke 5). These findings show that different levels of constriction in the brain extracellular environment have led many researchers to ask questions about ECS constriction. Their focus has mainly been before and during seizure activity. Here it is pertinent to mention the work of some researchers, such as McBain, Traynelis, and Dingledine (cited in Zhang & Koifman 2499), who have used high-K+ models on animals to answer these questions. Their findings have revealed that the ECS volume could reduce by up to 30% before the onset of a seizure (Zhang & Koifman 2499). They also highlight a direct correlation between cell mass increase and ECS constriction (Zhang & Koifman 2499). Experts have often strived to know the cell type that is responsible for the swelling, but researchers have provided little information to answer this question (Binder 247). Nonetheless, Binder says that “astrocytes but not neurons express aquaporin water channels that allow rapid facilitated water transport and swelling in response to osmotic gradients” (250). Relative to this statement, Beenhakker (612) says evidence has shown that osmotic processes often lead to the swelling of astrocytes and not their associated neurons. However, there is insufficient evidence surrounding the prediction of astrocyte or neuron swelling during the pre-ictal stage (Binder 247). Nonetheless, some independent research studies have hinted at pre-ictal calcium signalling before the onset of seizures (Arizona State University 8). Their findings suggest that astrocyte activation may occur before the onset of seizures. Modern instruments for predicting the onset of epilepsy often use such information to alert a patient of an impending seizure (Arizona State University 8).
System Design
For a long time, people always thought that epileptic seizures occurred randomly and there was no way of predicting its occurrence. While some people still hold this belief today, scientific advancements in medical research has proved otherwise (Scaramelli & Braga 246; Bethesda National Institute of Neurological Disorders and Stroke 1). These research studies have stemmed from episodes of patients reporting specific periods when they believed seizures were most likely to occur. However, they have been unable to specify dates or times when they would occur. This paper has capitalized on the need to predict seizures because the unpredictability of seizures could lead to fatalities or injuries, especially if the patients are alone, or are engaged in “life-threatening” activities, such as swimming or driving. The mobile epilepsy predictive system relies on EEG recordings to predict the onset of a seizure. Although there are still many issues regarding the applicability of EEG readings for this purpose, advancements in computer technology and digital EEG technology have allowed researchers to understand EEG recordings and put them to good use using mathematical algorithms to predict the onset of seizures (Scaramelli & Braga 246; Bethesda National Institute of Neurological Disorders and Stroke 1). Although this paper has shown that, the mobile epilepsy predictive system could predict seizures 40 minutes before it occurs, studies from the 1970s show that these seizures may occur for long periods (Dzhala 7873). Hemodynamic studies have brought a new twist to this assessment by showing that increased blood flow within the epileptic hippocampus is synonymous with the onset of seizures or their associated muscle convulsions (Fricker 559). To come up with accurate assessments for predicting seizures, it is prudent to have an effective set of algorithms for easy interpretation of EEG data.
Algorithms
Over several decades, different people have developed different sets of algorithms for predicting the onset of seizures. Most of the researchers who have developed them have done so retrospectively (Scaramelli & Braga 246; Bethesda National Institute of Neurological Disorders and Stroke 1). Stated differently, although people from different parts of the world have their unique versions of these algorithms, few of them have demonstrated clinical usefulness. According to Rajesh (19), this drawback is the biggest challenge associated with seizure prediction. It mainly stems from the inconsistencies associated with reading EEG data. Varied sensitivity and specificity of statistical testing methods have also compounded this problem because they have caused a lack of uniformity in reading EEG data (Scaramelli & Braga 246; Bethesda National Institute of Neurological Disorders and Stroke 1). Researchers have used several algorithms to improve the efficiency of the mobile epilepsy predictive system during the pre-ictal period (Bethesda National Institute of Neurological Disorders and Stroke 8). For example, the method used in the CHB-MIT database includes data from 22 patients, 22 EEG channels, and the number of seizures per patient (Haut 276). The number of seizures per patient emerges from using statistics from the pre-ictal, post-seizures and normal states of patients before they experience seizures. The methods for calculating the pre-ictal period also include a set of univariate time and frequency domain features (Haut 276). They are calculated on 5s time-chunks 264 features, which include 12 features and 22 channels (Haut 276). The pre-ictal period also uses data from cluster TCs by checking the number of TCs from each class (Bethesda National Institute of Neurological Disorders and Stroke 8). The classes comprise of the pre-ictal, inter-ictal, and seizure stages. The diagram below represents the holistic understanding of the pre-ictal period.
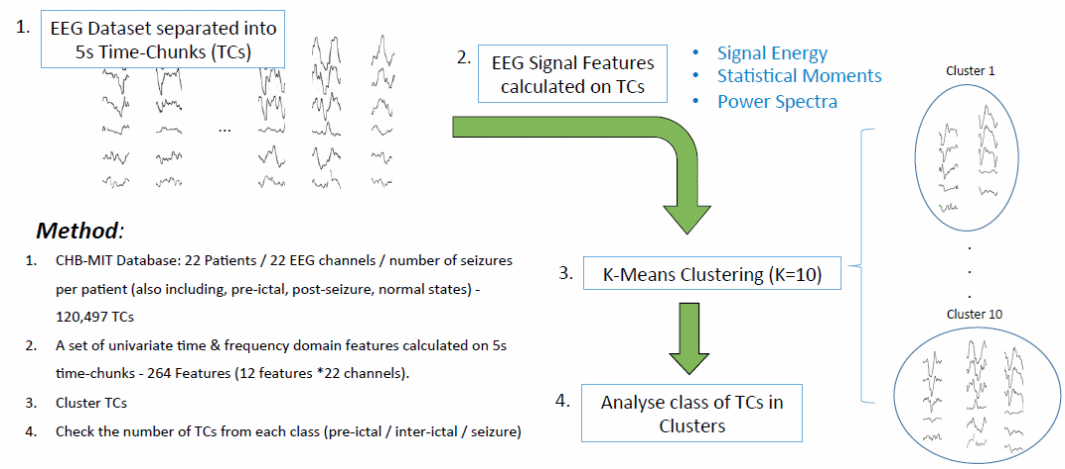
According to Lasemidis and Shiau (616), extraction and machine learning techniques have been instrumental tools for developing effective algorithms for managing epilepsy Bethesda National Institute of Neurological Disorders and Stroke (1) supports this narrative. Extracting the information obtained from EEG machines is a difficult process because it requires advanced methods for doing so. As such, Alotaiby and Alshebeili (18) say the use of neural networks and support network machines are effective tools for extracting information from EEG segments. Haut (276) adds that some researchers have started using Bayesian-based methods to predict seizures. The importance of using these methods and instruments comes from the complex nature of reading EERG signals. In line with this observation, Huang says, “EEG signals are spontaneous electrical brain activities that exhibit dynamic, stochastic, nonlinear, non-stationary and also complex behaviour” (59). Traditional time analysis techniques have also assumed a linear or stationery approach to the analysis of EEG data. However, new researchers who believe their techniques could provide a more effective way of reading EEG data have proposed new techniques. For example, Ozdemir and Yildirim (1) propose the use of the Hilbert-Huang transform (HHT) technique to process EEG signals. Their method is more accustomed to read non-liner and no-stationary information. This technique is not only a powerful tool for reading EEG signals because it can read ECG signals as well. According to Lipton, “A recent study was conducted on a method for implementation of EMD for computationally efficient and accurate real-time analysis and the method’s efficiency was shown for EEG and ECG datasets” (927).
Clustering Analysis
In an ideal state, it is easy to dominate each cluster of the algorithm by using one stat. However, this rarely happens because in many instances, there are many inter-ictal and pre-ictal TCs. This analysis shows that, given the current data and features outlined in the algorithm, it is difficult to ascertain the pre-ictal and inter-ictal states. Nonetheless, a learning procedure would help to do so (Ozdemir & Yildirim 1). The diagram below shows how different clusters appear through the inter-ictal and pre-ictal TCs.
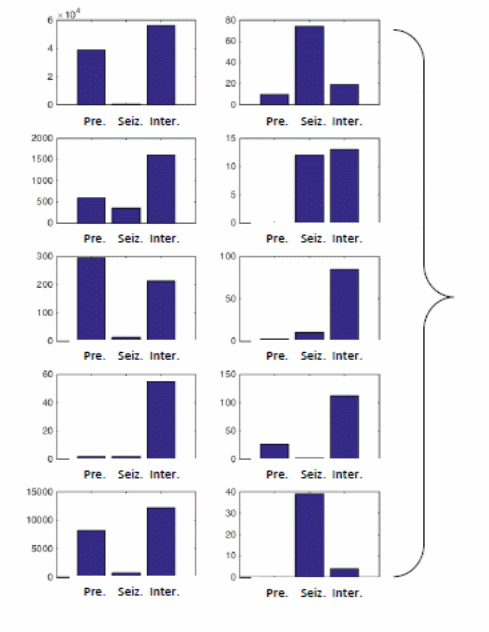
Based on the diagram above, the TCs emerging in each of the above cluster follow their respective classes. The length of the bar in each cluster depicts the number of TCs in each category.
The Pre-ictal Period
This paper has already shown that pre-ictal period refers to a patient’s status before he experiences a seizure. The method used to calculate data in the pre-ictal period is almost similar to the methods used to do the same in the working hypothesis segment. For example, the CHB-MIT database includes 22 patients, 22 EEG channels, and the number of seizures reported from each patient (Scaramelli & Braga 246; Bethesda National Institute of Neurological Disorders and Stroke 1). The number of seizures will include the pre-ictal, post-seizure, and normal states of every patient. Calculations in the system design process also include information from a set of univariate time and frequency domain features, which are calculated using 5s time-chunks. The 264 features emerge from multiplying 12 features and 22 channels (Scaramelli & Braga 246). The pre-ictal period also includes information from the random classifier, which are separate for every patient received. The method also includes leave-seizure-out cross-validation, which assess the accuracy of the prediction. The following diagram summarizes these processes
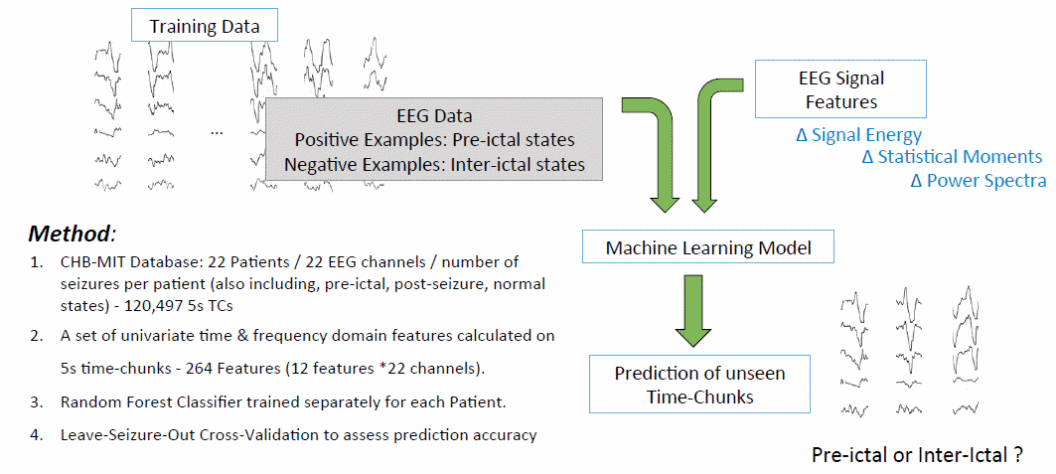
When exploiting multivariate causal information in the pre-ictal period, discriminating between the pre-octal and inter-ictal states require the exploration of two avenues. The first avenue is causality, which assesses the information flow in the pre-ictal state (Scaramelli & Braga 246; Bethesda National Institute of Neurological Disorders and Stroke 1). This stage uses causality inference methods (Granger causality). In this state, we are starting to build directed networks, which show information flow across the time and frequency domains (Lasemidis & Shiau 617). These domains cover both the pre-ictal and inter-ictal states. Using the flow of information described above, it is easy to develop network related features for shoring the distinctions between the pre-ictal and inter-ictal states (Lasemidis & Shiau 617). For example, information obtained from social networks and genetic networks could distinguish between pre-ictal and inter-ictal states (Lasemidis & Shiau 617).
Besides accounting for information from the pre-ictal state to distinguish between the inter-ictal and pre-ictal stages, another way of doing so is to account for clinical and physiological seizure variables (Scaramelli & Braga 247). By doing so, we would answer several questions surrounding the pre-ictal stage, such as understanding whether it exists or not. This way, we would also answer questions that are more fundamental to the development of the mobile epilepsy predictive system, such as understanding how the pre-ictal stages differ from different types of seizures detected using the mobile epilepsy predictive system. This need emerges from the difficulty of looking for general patterns during the pre-ictal stages (Scaramelli & Braga 246; Bethesda National Institute of Neurological Disorders and Stroke 1). Sackellares (58) says evaluating this need is important because there are different kinds of clinical seizures, which may occur because of different physiological factors. Through this analysis, the fundamental question we would be asking in this context is “how does the pre-ictal period change for different types of seizures?” In line with this narrative, it is important to understand that there are different categories of seizures that include partial seizures and generalized seizures (Scaramelli & Braga 246; Bethesda National Institute of Neurological Disorders and Stroke 1). Partial seizures often occur from localized sources. According to Schuyler (75), they often do not lead to the loss of consciousness because they are simple. However, in some cases, they may be complex and include a loss of consciousness. The second category of seizures includes general seizures that often emerge from non-localized sources. “Absence,” which manifests through daydreaming or sudden seizures that may take only a few seconds characterizes them (Scaramelli & Braga 246; Bethesda National Institute of Neurological Disorders and Stroke 1). Such seizures may also manifest through myoclonic effects, often characterized by sudden muscle jerks. Schuyler (75) also says that they are “tonic” because a patient’s muscles stiffen and they lose consciousness. However, sometimes, these cases may be atonic, where the muscles stiffen and the patient crumbles to the ground. These features may appear through the following steps
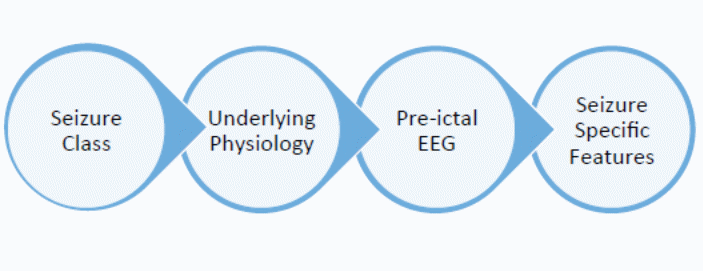
The process between the underlying physiology and the pre-ictal EEG shows how class and physiological differences often emerge through EEG data. The interlude between the seizure class and the underlying physiology denotes the process whereby health practitioners understand the physiology behind different classes of seizures (Scaramelli & Braga 246; Bethesda National Institute of Neurological Disorders and Stroke 1). Lastly, the stage between the pre-ictal EEG stage and the seizure specific features denote the process of mapping the EEG realizations into seizure-specific features. The following figure presents this flow
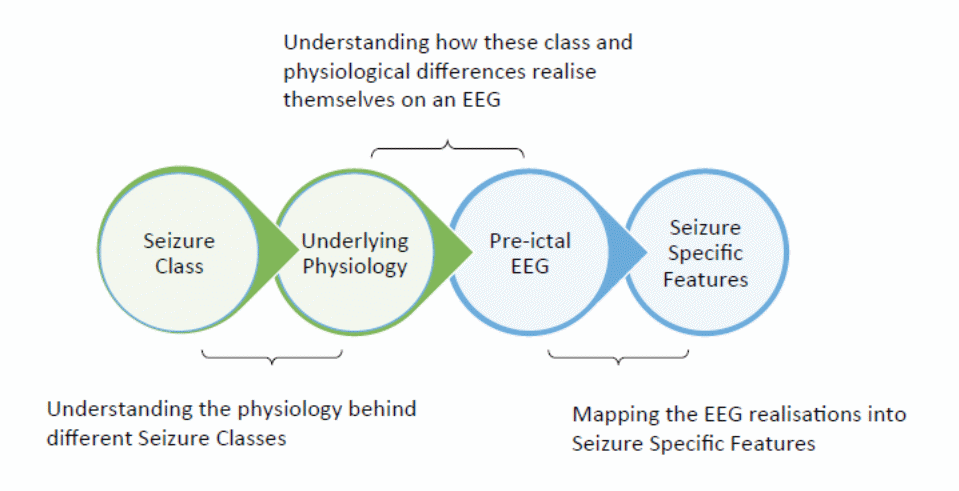
Collectively, the above steps account for clinical and physiological seizure variability.
The wireless predictive system highlighted in this paper should predict the occurrence of seizures among epileptic patients. With the aid of such a predictive system, patients could lead normal lives because they could minimize the possibility of injury of death during a seizure, or prevent its occurrence altogether (Scaramelli & Braga 246; Bethesda National Institute of Neurological Disorders and Stroke 1). Nonetheless, Eftekhar (47) insists that although such mechanisms may reduce the incidence of fatal seizures, it is still wise to make sure that a patient is not alone when a seizure occurs.
Many researchers have developed different types of technologies for reading signals from the patient’s body (Rajesh 1; Scaramelli & Braga 246; Bethesda National Institute of Neurological Disorders and Stroke 1). For example, many devices contain sensors that could detect abnormal brain activity, or involuntary muscle contractions that are synonymous to epilepsy. For example, pulse oximetry is an advanced technique used by researchers to measure a patient’s pulse rate. Using such a technique, patients could monitor their heart rates and know when there are abnormal activities. Micro electromechanical sensors (MEMS) are other mechanisms used by researchers to measure muscle contractions, as another predictive indicator of an imminent seizure (Scaramelli & Braga 246). The device is small and uses small sensors attached to a patient’s body to detect abnormal muscle movements. Observers say it is an accurate and precise instrument for detecting muscle contractions (Scaramelli & Braga 246).
To provide effective wireless tools for predicting seizures, it is important to provide wireless communication channels, such as the Mi-Wi protocol. The selected mobile epilepsy predictive system often uses Wi-Fi for this purpose. The MEMS signal has a good record in monitoring variations in a patient’s heartbeat rate. When the device detects abnormal activities, it relays the same to external devices for immediate remedial action (Scaramelli & Braga 246). The mobile epilepsy predictive system uses the same mechanism, except for the fact that it uses Wi-Fi to communicate bodily signals to electronic devices (Neapolitan 15). Besides mobile phones that relay epilepsy warnings, researchers have also used wearable electronic devices, such as watches and bracelets, to serve the same purpose (Scaramelli & Braga 246; Neapolitan 15). Recent advancements in technology have increased the device’s capability to make them environmentally safe for patients. This is why Huang says, “The device can sense the aura of pre-ictal stage in a few minutes and take the necessary safety measures automatically” (99). In this regard, patients do not require technical assistance when they have such devices. This advantage increases the appeal of such devices to young people who may be suffering from epilepsy, but want to live an active life. Indeed, the user gets to enjoy the benefits of having a wireless and portable device (properties that would allow them to live an uninhibited life). Based on these dynamics, implementing the mobile epilepsy predictive system would require several considerations. First, there should be a known criterion for sensing biometric signals. Although two biological signals are ideal in detecting abnormal body movements (heartbeat and muscular movements), the mobile epilepsy predictive system would mainly focus on using brain wave activities to predict the occurrence of a seizure (Scaramelli & Braga 246; Neapolitan 15). Nonetheless, Cohen (87) says there is nothing wrong with complementing this approach with the addition of a pulse oxymeter to measure heart rates and MEMS sensors for measuring muscle movements because they only add to the accuracy of predicting seizures.
Processing information and making decisions are also other considerations for implementing the mobile epilepsy predictive system. An electronic device (mostly a tablet, PC or smart-phone) should be able to do so (Rajesh 1). However, such devices should correctly know which signals relate to a seizure and which ones do not. Thirdly, there should be a communication medium for relaying biological signals to the electronic devices. This means that for the mobile epilepsy predictive system to work there should be a Wi-Fi signal. According to Rajesh (1), there should be a controlling mechanism for the patient to know of the presence of an imminent seizure. This addition means that all users should integrate an alarm device to the smart-phone to alert them of a seizure. Without such integrations, the patient would not understand what is happening, thereby rendering the entire apparatus useless (Rajesh 1; Scaramelli & Braga 246; Bethesda National Institute of Neurological Disorders and Stroke 1). Lastly, although using the mobile epilepsy predictive system is beneficial in detecting seizures before they happen, it has constraints that could limit their application. For example, patients should always be around places that have power because their mobile devices require frequent charging.
How the Mobile Epilepsy System differs from other types of Instruments
The mobile epilepsy predictive system differs from other types of instruments for predicting the occurrence of seizures because of its non-invasive design. For a long time, researchers have used invasive procedures that involve planting a device in the patient’s chest or head to detect the occurrence of a seizure (Rajesh 1; Scaramelli & Braga 246; Bethesda National Institute of Neurological Disorders and Stroke 1). For example, an Australian study recently did a study to integrate chest-implanted devices with the patient’s brain through electrodes that connect the two body parts (Scaramelli & Braga 246; Neapolitan 15). This method also used a handheld device that flashed “red” to warn the patient of an imminent seizure. Its efficacy level was 65% (Scaramelli & Braga 246; Neapolitan 15). However, the main drawback of this method was its failure to cater to patients’ individual needs. This is why proponents of the system are still trying to understand the best patient profile that could use the system.
Other Types of Seizure Prediction Systems
Global Seizure Prediction System
The global seizure prediction model is a one-size-fits-all model. It has a less complex implementation process and it is similarly difficult to account for patient variability. These features make it less desirable for managing epilepsy because the mobile epilepsy predictive system is more effective in managing epilepsy among anomalous patients (Rajesh 1; Scaramelli & Braga 246; Bethesda National Institute of Neurological Disorders and Stroke 1). The global seizure predictive system also distinguishes itself from other types of predictive systems because it uses offline data sets. Stated differently, there is no use for data from the actual patient. The global seizure prediction system is often synonymous with the adaptive seizure prediction algorithm (ASPA). Unlike researchers who believe that the global seizure prediction system does not have a high efficacy rate, studies conducted by Arizona State University (55) reveal that it could predict up to 80% of seizures. It only has a false prediction rate of 0.16 per hour (Arizona State University 55). In fact, unlike the mobile epilepsy predictive system, which can predict seizures 40 minutes before they happen, Beenhakker (615) argues that the global seizure prediction system could predict a seizure, 70 minutes before it happens. This efficacy shows that medical researchers could use the global seizure prediction system to develop therapies (Rajesh 1; Scaramelli & Braga 246). Similarly, its reliability is useful in developing and using implantable devices to predict the onset of seizures. Although researchers have synonymously used the ASPA in global seizure prediction systems, Lopantsev (357) reveals that there have been improvements made in its application through improved seizure prediction algorithms. These improvements emerge from spatial synchronizations of all entrained pairs of sites.
Individual Seizure Prediction System
Details emerging in this study show that the mobile epilepsy predictive system relies on a set of algorithms to predict the onset of a seizure. These algorithms often use different sets of information to predict when a seizure will occur, such as the assessment of the seizure occurring and the EEG recordings (Rajesh 1; Scaramelli & Braga 246; Bethesda National Institute of Neurological Disorders and Stroke 1). These pieces of information are reliable measures of a person’s brain activity before the onset of seizures. However, they are abstract and inapplicable in developing patient-specific instruments for seizure detection. For example, Carney (94) says that most experts cannot use such information (reliably) to develop implantable devices, or to develop individual therapies. The importance of having a patient-specific seizure detection technique stems from the use of time-domain methods to predict when seizures would occur (Rajesh 1; Scaramelli & Braga 246; Bethesda National Institute of Neurological Disorders and Stroke 1). This method shows that different patients have different forms of brain wave lengths for predicting the onset of seizures. Using this understanding, Schuyler (75) argues that seizure detection and prediction algorithms should be sensitive to the differences noted across different types of patients. The time domain technique is one among many other types of seizure detection methods. The diagram below will place it among its peers.
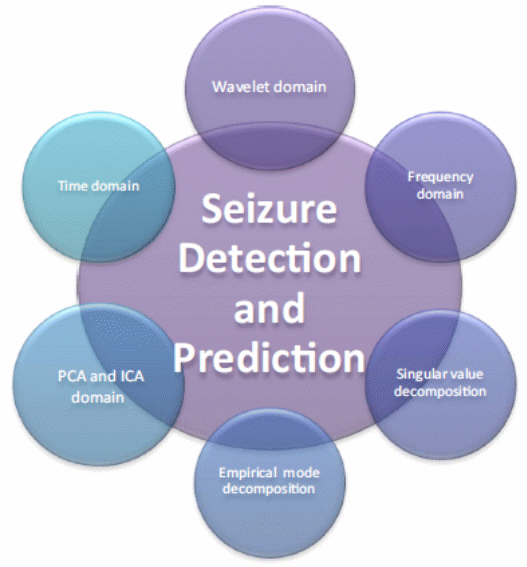
The above diagram shows that wavelet domain, frequency domain, singular value decomposition, empirical mode decomposition, and PCA domain are other seizure prediction methods. Different patients are bound to report different statistics for ictal prediction using any of the above-mentioned methods. This is why the individual seizure prediction method is important in developing sensitive EEG prediction instruments.
As its name suggests, the individual seizure prediction system is tailor-made to suit only one patient. The individual seizure prediction system is important in predicting epileptic seizures because, as the Bethesda National Institute of Neurological Disorders and Stroke (10) suggests, different patients have different EEG Signals. It has a complex implementation process that uses data from actual patients using the system, unlike the general system, which does not need data from actual patients. The individual seizure prediction system can also account for patient variability because the accuracy for the system’s information for each patient is unlimited. Stated differently, it is easy to retrain on new EEG data that comes in the prediction system.
Type-Specific Seizure Prediction System
The type-specific seizure prediction system is different from other forms of epilepsy predictive systems because it is tailor-made for specific types of seizures (Rajesh 1; Scaramelli & Braga 246; Bethesda National Institute of Neurological Disorders and Stroke 1). Using this predictive system requires clearly annotated datasets to use during training. Similarly, it is easy to learn about a patient’s medical history offline. Stated differently, the need for data from actual patients is low. The mobile epilepsy predictive system also uses such results to understand the different characteristics of different seizure types.
Summary
Despite the problems highlighted by some researchers surrounding the reading of EEG data, there are many benefits associated with an early warning system such as the mobile epilepsy predictive system (Scaramelli & Braga 246; Bethesda National Institute of Neurological Disorders and Stroke 1). Already this paper has shown that such a system could warn patients and caregivers about an imminent seizure and help them to take precautionary actions to minimize the risk of injury. This paper has also explained how the mobile predictive system works. It has also compared this predictive system with other types of systems that manage epilepsy. In particular, it has compared it to the type-specific seizure predictive system, the individual seizure predictive system, and the global seizure predictive system. An ideal mobile epileptic predictive system should be non-invasive and monitor a patient’s epileptic state, constantly. It should also suit individual needs because different epileptic patients have different types of needs. The mobile epilepsy predictive system has many of these characteristics. Its usefulness lies on the efficiency of its evolving prediction algorithm, which uses new EEG data and feeds it back to the system for retraining. This algorithm is sensitive to different states of the patient. For example, it could use information about a patient’s sleeping patterns, frequency of anger conversational exchanges, and exercising patterns to develop a new algorithm for monitoring a patient’s epileptic state. Indeed, different states would evoke different sub-algorithms to predict different epileptic states. The seizure prediction and suppression ability of the mobile epilepsy predictive system also makes the system unique to other types of predictive systems because it could turn on the seizure prediction system for high TP rates because FP is not an issue.
Lastly, smart-phones are not new additions to the management of epileptic seizures because patients have used them in the past to know how well their medicines are working (Rajesh 1; Scaramelli & Braga 246; Bethesda National Institute of Neurological Disorders and Stroke 1). In line with the goal of using smart-phones to predict when seizures would occur, researchers have learned to integrate them with the EEG technology. For example, dialog is a new app that has emerged from this push because experts have learned that it could help patients to get a better handle of their condition. By using this app, patients could know when a seizure would occur and take the appropriate actions to prepare for it. The mobile epilepsy predictive system outlined in this chapter uses the same mechanism to predict the onset of a seizure.
The Future of Epilepsy Prediction
The electronic system highlighted in this paper is a portable device that could alert a patient about the possibility of a seizure occurring, 40 minutes before it does. The device works by detecting and interpreting brain waves to know when a seizure is likely to occur. When the device detects abnormal brain activity, it sends a signal to an electronic device, which later relays the same to a digital mobile device, which alerts a patient about its occurrence. The coded signal detected from the brain is often decoded using a wireless device that pairs with a smart-phone. Future developments of this device should focus on creating a GPS device to pinpoint a patient’s location (Scaramelli & Braga 246; Bethesda National Institute of Neurological Disorders and Stroke 1).
Although this paper shows the potential for detecting seizures before they happen, using the mobile epilepsy predictive system, the field of research underlying its use is still underdeveloped. Therefore, currently, many devices in the market include mechanisms that could help patients to get help when they experience a seizure, but they cannot properly detect one, at least accurately, before it happens. Nonetheless, the development of an effective and accurate epilepsy predictive system would help patients to prevent potentially harmful incidents that would occur when a seizure occurs. In fact, there is a high probability that the advancement of such methods could help to prevent a seizure, entirely, before it happens because patients could take medications to prevent their occurrence. Nonetheless, it is pivotal to understand that most of the technologies highlighted in this paper are still under development and it may take a long time before researchers produce instruments that could effectively predict seizures before they happen. Meanwhile, it may be beneficial for patient to follow their doctors’ treatment regimen.
References
Alotaiby, Turkey and Alshebeili Saleh. “EEG Seizure Detection and Prediction Algorithms: A Survey.” EURASIP Journal on Advances in Signal processing 13.1 (2014): 1-21. Print.
Amy, Norton. Implanted Device May Predict Epilepsy Seizures. 2005.
Arizona State University. Spatiotemporal Brain Dynamics in Epilepsy: Application to Seizure Prediction and Focus Localization, New York, NY: ProQuest, 2008. Print.
Beenhakker, Huguenard. “Neurons that fire together also conspire together: is normal sleep circuitry hijacked to generate epilepsy?” Neuron 62.1 (2009): 612–632. Print.
Bethesda National Institute of Neurological Disorders and Stroke. Curing the Epilepsies: The Promise of Research. 2013. Web.
Binder, Devin. “Toward new paradigms of seizure detection.” Epilepsy Behav 26.3 (2013): 247–252. Print.
Carney, Paul. “Seizure Prediction: Methods.” Epilepsy Behav 22.1 (2011): 94–101. Print.
Cohen, Lauren. Time-frequency Analysis, Englewood Cliffs, NJ: Prentice Hall, 1995. Print.
Drury, Smith. “Seizure prediction using scalp electroencephalogram.” Exp Neurol 184.1 (2003): 9–18. Print.
Dzhala, Staley. “Transition from interictal to ictal activity in limbic networks in vitro.” J Neurosci 23.1 (2003): 7873–7880. Print.
Eftekhar, Toumazou. “Empirical mode decomposition: real-time implementation and applications.” Journal of Signal Processing Systems 73.1 (2013): 43–58. Print.
Elsevier. People With Epilepsy Can Benefit From Smartphone Apps To Manage Their Condition. 2015. Web.
Fricker, Miles. “EPSP amplification and the precision of spike timing in hippocampal neurons.” Neuron 28.19 (2000): 559–569. Print.
Haut, Sheryl. “Predicting Seizures: Are We There Yet?” Epilepsy Curr, 13.6 (2013): 276–278. Print.
Huang, Long. “The mechanism for frequency downshift in nonlinear wave evolution.” Advances in Applied Mechanics 32.1 (1996): 59–117. Print.
Hughes, Devinsky and Feldmann Bromfield. “Premonitory symptoms in epilepsy.” Seizure 2.1 (1993): 201–203. Print.
Lasemidis, Leon and Shiau Deng-Shan. “Adaptive Epileptic Seizure Prediction System.” Transactions On Biomedical Engineering, 50.5 (2003): 616-627. Print.
Lipton, Bruce. “Predicting seizures: A behavioral approach.” Neurol Clinics 27.1 (2009): 925–940. Print.
Litt, Echauz.”Prediction of epileptic seizures.” Lancet Neurol 5.1 (2002): 22–30. Print.
Lopantsev, Avoli. “Participation of GABAA-mediated inhibition in ictallike discharges in the rat entorhinal cortex.” J Neurophysio 79.1 (1998): 352–360. Print.
Neapolitan, Ronald. Learning Bayesian Networks, London, UK: Prentice Hall, 2003. Print.
Ozdemir, Nilufer and Yildirim Esen. “Patient Specific Seizure Prediction System Using Hilbert Spectrum and Bayesian Networks Classifiers.” Computational and Mathematical Methods in Medicine 3.1 (2014): 1-10. Print.
Rajesh, Remanan. Smart Epilepsy Prediction And Life Saver System. 2013. Web.
Reynolds, Jerremy. Epilepsy: its symptoms, treatment and relation to other chronic convulsive diseases, London, UK John Churchill, 1861. Print.
Sackellares, Chris. “Seizure prediction.” Epilepsy Currents 8.3 (2003): 55–59. Print.
Scaramelli, Alejandro and Braga Patricia. “Prodromal symptoms in epileptic patients: Clinical characterization of the pre-ictal phase.” European Journal of Epilepsy 18.4 (2009): 246–250. Print.
Schulze-Bonhage, Feldwisch-Drentrup. “The role of high-quality EEG databases in the improvement and assessment of seizure prediction methods.” Epilepsy Behav 22.1 (2011): 88–93. Print.
Schuyler, White. “Epileptic seizure detection.” IEEE Engineering in Medicine and Biology 26.2 (2007): 74–81. Print.
Shiau, Iasemidis. “Adaptive epileptic seizure prediction system.” IEEE Trans Biomed Eng 50.5 (2003): 616-27. Print.
Watson, Stephanie. Bracelets and Devices for Epilepsy. 2014. Web.
Zhang, Zhang and Koifman Julius. “Transition to Seizure: Ictal Discharge Is Preceded by Exhausted Presynaptic GABA Release in the Hippocampal CA3 Region.” The Journal of Neuroscience 32.7 (2012): 2499-2512. Print.